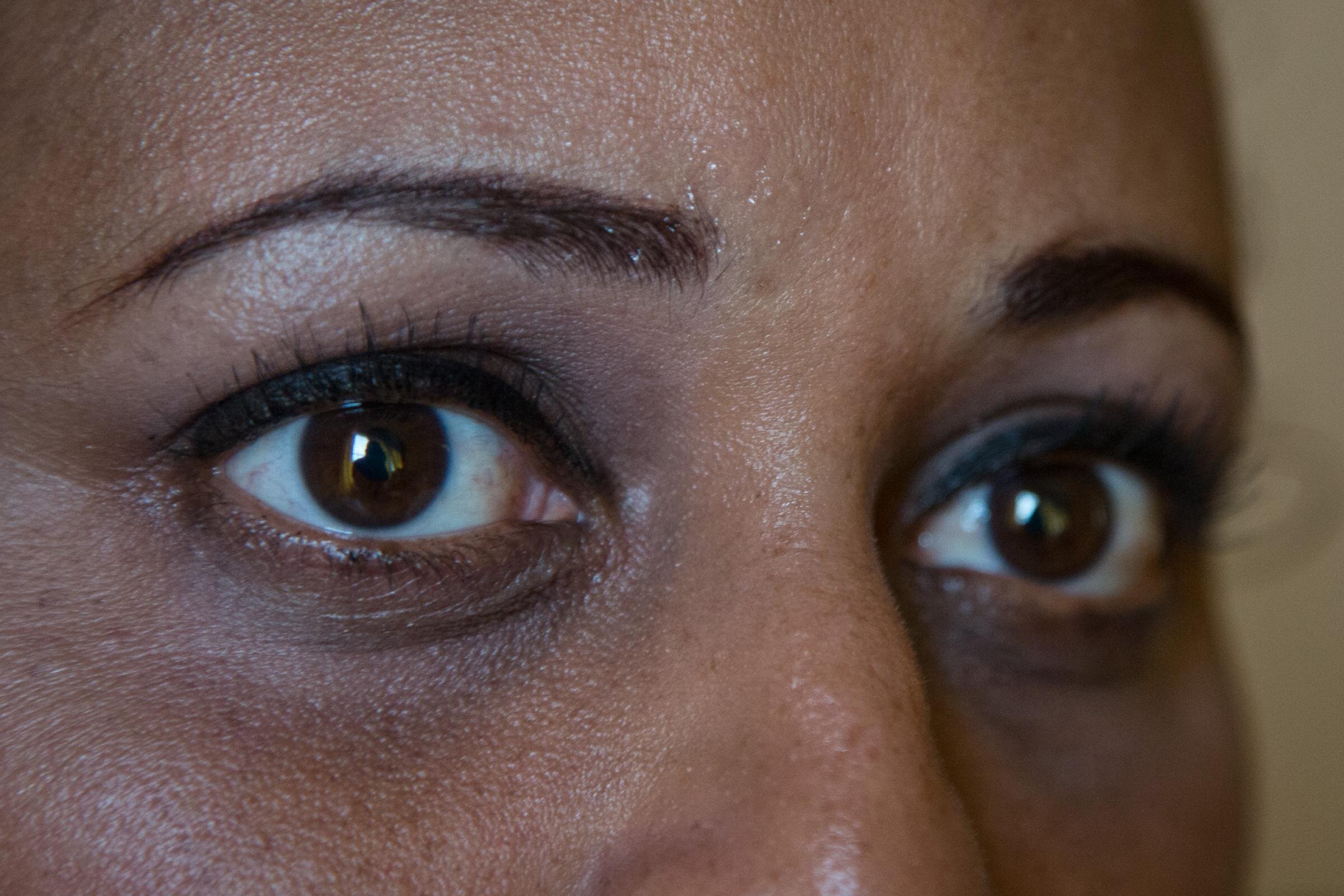
No Place Like Home
A Global Exploration of Violence Between Partners
An Investigative Report By: Neal Rothleder, Journalist, Email, Heather Krause, Chief Data Scientist, Email
CHAPTER 1
ALL ABOUT THE DATA
INTRODUCTION
Orb Media does journalism differently. We designed our story development process to be a true collaboration between data analysis and professional, on the-ground-reporting. This story, “No Place Like Home,” is our first effort in this redesigned method.
While the narrative text, audio and multimedia versions of “No Place Like Home” have some of the takeaways from Orb Media’s own data analysis woven into their fabric, we also wanted to provide a “data version” of the story. To that end, in this section you will find information about the data that was used, how it was used, as well as charts and text on the most interesting findings all together in one place.
There are a few things we hope to achieve by having data analysis and reporting work in conjunction to produce a story. First, we selected datasets that address issues related to domestic violence on a global scale and analyzed that data in order to facilitate our journalists’ exploration of questions around the issue. We seek to turn the data into something that can be treated as an informed source by our editorial team.
Second, we want to leverage the credibility and quality of existing studies and data sources to provide a useful context for understanding some of global characteristics of Intimate Partner Violence (or IPV). We want to emphasize that the goal is context and understanding, not research-oriented mathematical deep-dives. Third, we want the output of this work, our data products, to provide a demonstration of initial capability – establishing a launch pad for others to build upon and advance.
And finally, in an effort to bring some new insight into the issue of domestic violence, our data team looked more deeply into a few of the selected datasets that have not been explored extensively before. In particular, we took a data-driven look at the global connection between the prevalence rates of intimate-partner violence and the presence of laws that address it. And in the case of Uganda, we looked at changes over time in survey responses regarding intimate partner violence.
Domestic violence is a complex issue, and it is described differently around the world. For this project, Orb Media uses the phrases Intimate Partner Violence (IPV), Domestic Violence and Partner Violence interchangeably to describe physical or sexual violence between adults in an intimate relationship.
While the narrative versions of “No Place Like Home” weave the data products and human experience together to bring you a rich, multi-faceted story, this section is designed to give you more information, specifically about the data we worked with, how we worked with it and some of the interesting findings from that work. Some of what is in this section is designed for people who have a working knowledge of data analytics, but we hope that the curious will also explore it.
In the interest of transparency we provide the following in the chapters that follow:
A list of the original data sources we leveraged, with links.
A description of the cleaning and preparation process we used.
A discussion around the appropriate use of data processed in this way.
A larger sample of the analytic results we discovered.
Finally, we hope a wider community will build on our initial analysis, so we’ve also provided the cleaned datasets for download.
CHAPTER 2
ORIGINAL DATA SOURCES
ORIGINAL DATA SOURCES
While we started with a broad exploration of datasets, we eventually chose eight to support the reporting of this story:
USAID StatCompiler Data - USAID, Demographic and Health Surveys (DHS) STATcompiler. 2000-2011 (Varies by country).
EU VAW Data - Violence Against Women: An EU Wide Study. 2012.
OECD GID Data - Organisation for Economic Co-operation and Development - Gender, Institutions and Development Database. 2009 & 2012.
UN POWW Data - UN WOMEN - Progress of the World's Women. 2011-2012.
UN VAW Data - Violence against Women Prevalence Data: Surveys by Country. 2006 & 2011 (Varies by country).
WBL Data - World Bank - Women, Business and the Law. 2010 & 2012.
TweetWorld Bank DV Data - World Bank, Social Development - Domestic Violence Dataset. 1982-2007 (Varies by country).
Uganda DHS Data – USAID Demographic and Health Survey – Uganda Datasets 2000/2001, 2006 and 2011.
CHAPTER 3
CONSTRUCTING DATASETS FOR ANALYSIS
DATA PREPARATION AND SELECTION
Following an initial research and exploration period, each of the datasets listed in our Original Data Sources chapter was cleaned and processed in the following way:
The subsets of indicator variables that were relevant for our analysis were chosen.
Empty or missing values were left empty. Guidance from the documentation from the individual sources was used to interpret these values, but no imputation or inference was done.
Standard country names were applied so that datasets could be easily merged or compared.
In this way, all original data values were preserved. Our efforts were aimed at cleaning and standardizing values to facilitate analysis.
SPECIFICS ABOUT DATA TRANSFORMATION AND INTEGRATION
Data on IPV is often collected with reference to the types of violence (Physical, Sexual or Overall -- which includes both physical and sexual) and time frames (Ever Experienced or Experienced in the Last Year). After analyzing the different combinations, we decided to combine indicators for Overall IPV Ever with Physical IPV Ever (most countries had data for only one of those) to create a single “IPV Prevalence Ever Experienced” indicator with broad global coverage. When multiple sources have data for the same country and the same year, we prioritize the sources. For this story we excluded ‘Sexual IPV’ indicators and ‘Experienced IPV in the Last Year’ indicators from our analysis.
As a result, we use 81 counts of “IPV Overall Ever Experienced” and 111 counts of “IPV Physical Ever Experienced” and when those are combined (giving preference to the IPV Overall Ever data) it gives us IPV prevalence data for 115 countries. When we further combine that data with the dataset that addresses laws on domestic violence, this produces a transformed dataset covering IPV prevalence and the countries that have laws for 95 countries. Of those 95, we only have data on attitudes towards intimate partner violence for 36 countries. Please see below for more details on these transformations.
We combined legislation data from two sources. The OECD GID data on IPV laws only covers developing countries. The UN POWW data also includes IPV law information, and includes both developing and developed countries. Note that this data does not specify when the laws were passed – just whether or not there were laws in place at the time of the data collection. For the OECD GID dataset, the Domestic Violence law indicators were transformed to a binary value by rounding values above 0.5 to 1 and values 0.5 and below to 0. There is a small bit of extrapolation done when completing data gaps in years without law data. Where there is surrounding data on both sides of the year that are the same, the missing value was added (E.g., A “yes” in 2010 and a “yes” in 2012 is extrapolated to “yes” in 2011).
A key component in understanding domestic violence is discerning the attitudes that people – both women and men – have about gender roles, rights and responsibilities. Many research efforts and surveys construct specific questions and indicators around this topic. Example topics include, “Is a wife obligated to have sex with her husband?” and “Is it acceptable for a husband to beat his wife if she refuses to have sex with him?”. We used two sources of data on IPV attitudes. The first is the USAID StatCompiler data, which captures many different circumstances (e.g., burning the food, or refusing to have sex), and the corresponding percentage of women who respond on the acceptability for a husband to beat his wife. This data is available for a range of countries and years. The second source of attitude data is the OECD GID data. This captures the overall population rate of women who generally believe that it is acceptable for a husband to beat his wife. This data is all from 2012.
The OECD GID data contains lifetime prevalence rates of IPV as well as a number of socioeconomic and demographic variables. It includes only developing countries, and for the year 2012. The WBL data includes both developing and developed countries. It includes a number of interesting variables concerning women’s equality and has data from 2010 and 2012.
When we combine the Socio-Economic Indicators, Social Attitudes and Legislation data with the IPV Prevalence data described above, the vast majority of the resulting dataset is 2012, with a few countries using 2010 data.
In exploring the data for “No Place Like Home”, we wanted to look at the global picture, but to also take a deeper dive into one country. By doing this we hoped to understand the insights a more focused look at a more detailed dataset (not widely available at a global scale) would yield. The Demographic and Health Surveys for Uganda contain hundreds of variables; these variables include individual-level microdata from a representative sample of adult men and women. A selection of these variables relate to experiences of and attitudes towards intimate partner violence as well as various socioeconomic factors. Each of the survey questions designed to capture data about the prevalence of and attitudes towards domestic violence in specific circumstances were combined into binary indicators in order to evaluate prevalence and attitudes towards domestic violence in any of the specified circumstances.
We collected data from 3 years of surveys (2000/2001, 2006, 2011), and created a separate dataset for each - containing the derived prevalence and attitude indicators along with selected socio-economic indicators.
CHAPTER 4
APPROPRIATE USE OF DATA
DATA ANALYSIS
As Orb Media begins to push the boundaries of integrating data analysis with professional journalism, we are exploring the range of data analytic investment - from “rigorous” (i.e., academic quality, which involves a great deal of time, energy, and resources) on one hand to “good enough” (in which the work conforms to accepted practices) on the other hand. We want to fall comfortably in the middle – with our emphasis on sharing the story of the data and enlightening our audience. There are always cautions and caveats when dealing with data analysis, and we want to be very clear about the limitations of what our data team was able to do in this initial foray. Towards that end, here is an important list of caveats regarding our analysis:
This is a descriptive analysis designed to summarize what broad sets of data tell us. We are trying to paint a picture across the globe to give context. Our objectives are a bit different from those trying to discover new scientific theorems or publish papers in academic journals.
There is no global, master data source. Most of the data captured in this analysis was collected via different means depending on the country. It includes surveys from different organizations, with different coverage and sample size, as well as different study population and age groups. Care must be taken in drawing conclusions from disparate datasets such as this.
Due to the nature of our data – merging and comparing a variety of datasets to get a high-level, global picture – in order to be useful at this stage, our analysis only explores pairs of variables. Existing research shows that there are many interacting effects and confounding variables within the complex world of domestic violence. We do not attempt to account for this. Thus, we cannot conclude a cause-and-effect relationship among the factors we connect. We merely conclude that the two factors have some correlation – they are connected by some set of relationships.
Due to the nature of the subject, IPV survey data (on which we rely heavily) is prone to bias and under-reporting.
CHAPTER 5
HIGHLIGHTS AND INTERESTING FINDINGS
GLOBAL PREVALENCE
IPV is truly a global issue. The map below shows prevalence rates for IPV for 115 countries.
In the national studies we analyzed, the IPV rate can be over 70 percent of women.
Among those with the highest survey rates of IPV are Angola, Bangladesh and Kiribati.
Among those with lowest survey rates of IPV include Armenia, Georgia, Morocco and Switzerland.
PREVALENCE AND LAWS
Both the OECD GID and the UN POWW data capture and code for the existence of laws specifically targeting domestic violence. The map below shows our transformed data – indicating for each country the presence or absence of laws in the most recent year for which we have data. Of the 203 countries we have law data for, 123 have laws relating to domestic violence.
Intuition would suggest that countries with IPV-related legislation would have lower IPV rates. Conversely, countries with no such laws would have higher rates.
The bar chart, below, allows us to readily see high and low IPV prevalence rates and their corresponding law status. The height of the bars indicates the IPV Prevalence Rate, with the color of the bars indicating presence/absence of anti-IPV laws.
Of Interest:
When looking at overall trends, the countries with IPV laws have, on average, a 5 percent lower IPV rate than those countries without laws.
This is statistically significant when tested through Pearson’s correlations and regression models. Wald tests were used within the regression models to determine significance.
There are quite a few counter-examples, however.
For example, both Austria and Armenia have relatively low rates of IPV, yet neither have IPV related legislation.
Uganda and Bangladesh both have IPV related laws, and yet both have among the highest rates of IPV.
We do not have sufficient data to support the question of how a change in laws affects the IPV rate in the country. We cannot say whether or not this relationship happens.
THERE ARE QUITE A FEW COUNTER-EXAMPLES, HOWEVER. FOR EXAMPLE, UGANDA (59 PERCENT) PASSED A LAW CRIMINALIZING DOMESTIC VIOLENCE IN 2010, AND IT HAS A HIGHER THAN AVERAGE RATE OF DOMESTIC VIOLENCE. THIS CHART ALLOWS US TO READILY SEE HIGH AND LOW INTIMATE PARTNER VIOLENCE RATES AND THEIR CORRESPONDING LAW STATUS.
PREVALENCE AND ATTITUDES
Overall, countries where more women say they find intimate partner violence acceptable are also countries where more women experience IPV. A 10 percent increase in IPV acceptance by women is associated with a corresponding 3.8 percent increase in IPV prevalence. (This is significant with a Wald test result of F=12.81 with a p-value of 0.0011)
This chart plots attitudes towards IPV against prevalence of IPV. Each colored spot represents an individual country. The horizontal axis represents the percentage of women in the country who find IPV acceptable. The vertical axis represents the percentage of women in the country who have experienced IPV. The line shows the statistical relationship between the two variables. While the relationship is significant, the plot shows a great deal of variation in this pattern and there are many exceptions and outliers to the general relationship.
A particularly large effect appears between IPV rates and attitudes involving women refusing to have sex with their husbands. Countries in which more women agree that a husband is justified in beating his wife if she refuses to have sex with him have a 6 percent to 7 percent higher average rate of IPV than countries that have a lower such agreement rate.
More generally, a 10 percent increase in the percentage of women who believe it’s acceptable for a husband to beat his wife in the following situations corresponds to an associated increase in IPV rates (average across countries) of:
Burns the food: 4 to 6 percent
Argues with him: 2 to 4 percent
Goes out without telling him: 1 to 3 percent
Neglects the children: 2 to 3 percent
Refuses to have sex with him: 6 to 7 percent
These estimates are derived from a regression model that includes the IPV prevalence rate as the dependent variable and the attitude data as the independent variable. We included controls for year. The prediction is made using Wald tests and a prediction matrix.
The bar chart below represents “IPV Prevalence Rates by Percent of Women Who Think it is Acceptable for a Man to Beat his Wife if She Burns the Food.”
Each country has two bars:
One bar shows the percentage of women in the country who think it is acceptable for a man to beat his wife if she burns the food.
The other bar shows the percentage of women in the country who have experienced IPV.
PREVALENCE AND INCOME
The OECD GID dataset assigns countries into one of four income groups: Low, Low Middle, Upper Middle or High. The map below captures this assignment for countries where we have such data.
The OECD GID dataset assigns countries into an income group: Low, Low Middle, Upper Middle, High.
Countries that fall into the "low income" category have a higher average rate of IPV than other countries.
This was tested with a factorial regression of income group on IPV rates. The Income Group designation is statistically significant in predicting IPV rates. This is tested through a Wald test with an F statistic of 4.6 on 3/53 degrees of freedom with a resulting p-value of 0.006.
In the figure below, the height of the bar indicates the average IPV rate for countries in each Income Group.
PREVALENCE AND CUSTOMARY LAWS
Some countries recognize customary law -- be it religious, tribal or cultural practice -- as a valid source of law under the constitution. The WBL data captures this through a set of conditions. The map below captures the status of these recognitions for countries where we have such data.
In 2012, countries that recognized customary law as valid under the constitution had a significantly higher average IPV rates than those countries that do not.
This was tested with a factorial regression of income group on IPV rates. The Customary Law status is statistically significant in predicting IPV rates. This is tested through a Wald test with a F statistic of 34.5 on 1/73 degrees of freedom with a resulting p-value of <.001.
In the figure below, the height of the bar indicates the average IPV rate for countries in each group.
For data from 2010 the difference between the two groups was not statistically significant (and also based on very little data).
PREVALENCE AND GENDER EQUALITY
Within the OECD GID dataset, we focused on 13 indicators capturing gender equality for women.
Age of marriage
Parental authority
Parental authority after divorce
System of inheritance
Son preference
Access to land
Access to credit
Access to public space
Political quotas
Percentage of women between the ages of 15 and 19 years old who are married
Percentage of women finding IPV acceptable
Percentage of last children that are male
Percentage of women in parliament
Of these 13 indicators, 11 had a straightforward, statistically significant relationship with the Overall Lifetime IPV. (“Access to public space” and “Political quotas” were the exceptions.) Generally speaking, among these indicators for these countries, more equality for women is associated with lower IPV rates.
Among those 11 variables, we highlighted two in the next findings.
In the OECD GID dataset, women’s access to credit is captured as one of three values:
Women have no access to credit
Women have access to some credit or access that is restricted
Women have the same access to credit as men
The map below captures the status of this grouping for countries where we have such data.
Note that in Nicaragua, women have equal access to credit as men, while in Uganda, women have no access to credit.
WOMEN’S ACCESS TO CREDIT
Countries in which women have more equal access to credit have a lower average IPV rate than those with less equal access to credit.
This was tested with a factorial regression of income group on IPV rates. The Access to Credit Level is statistically significant in predicting IPV rates.
This is tested through a Wald test with a F statistic of 154.0 on 3/53 degrees of freedom with a resulting p-value of <.001.
This is one of the strongest correlations among the gender equality variables.
In the figure below, the height of the bar indicates the average IPV rate for countries in each group.
POWER. MONEY. WHEN WOMEN’S ACCESS TO CREDIT IS EQUAL TO MEN’S COUNTRIES HAVE LOWER PREVALENCE RATES OF DOMESTIC VIOLENCE.
WOMEN’S ACCESS TO LAND
In the OECD GID dataset, women’s access to land is explored through, “women’s legal rights and de facto rights to own and/or access agricultural land.” Their access to land is captured as one of three values:
Women have no access to land
Women have mixed equity in access to land
Women have equal access to land as men
The map below captures the status of this for countries where we have such data.
Note that in Nicaragua, women have mixed equity in land access and in Uganda women have no access to land.
Countries in which women have more equal access to land have a lower average IPV rate.
This was tested with a factorial regression of land access group on IPV rates. The Access to Land Level is statistically significant in predicting IPV rates. This is tested through a Wald test with an F statistic of 5.8 on 3/53 degrees of freedom with a resulting p-value of .01.
In the figure below, the height of the bar indicates the average IPV rate for countries in each group.
POWER. MONEY. LAND. THE THREE ARE DEEPLY INTERTWINED. CASE IN POINT: COUNTRIES WHERE WOMEN HAVE EQUAL ACCESS AS MEN TO AGRICULTURAL LAND OWNERSHIP HAVE LOWER RATES OF DOMESTIC VIOLENCE. NICARAGUA IS DESCRIBED AS “WOMEN HAVE MIXED ACCESS TO LAND” AND UGANDA AS “WOMEN HAVE NO REAL ACCESS TO LAND.”
INTIMATE PARTNER VIOLENCE IN UGANDA
During the initial data exploration in which our journalists decided which countries to visit, the data team did a few country-specific data profiles. Here are some of the interesting highlights regarding Uganda.
The DHS Program is sponsored by USAID and gatherers globally harmonized data through the Demographic and Health Surveys. The organization collects, analyzes and disseminates country-level representative data through more than 300 surveys in over 90 countries. We used the Uganda DHS general surveys from three years: 2000/2001, 2006 and 2011. While individual years have been studied and reported on, limited work has been done looking at multiple years, i.e., at the changes over time. That is one place on which we wanted “No Place Like Home” to shed some light. In particular, we focused on changes in responses between the 2006 and 2011 surveys.
Overall, the prevalence rates did not change on a population level between 2006 and 2011.
In 2006 approximately 59 percent of women responded that they had experienced IPV. In 2011 approximately 60 percent of women responded that they had experienced IPV.
This apparent 1 percent change is not statistically significant.
Over time, both men and women are becoming less accepting of violence against women. Overall, fewer women approve of IPV over time. The largest change in attitudes occurs between 2006 and 2011, and appears in the table, below.
The difference in attitudes between the 3 years is statistically significant: Chi-Square = 807.1 on 6 df has a p-value of less than 0.001.
The bar chart captures the relationships described – making clear the idea that while attitudes about IPV are improving, the actual prevalence rate is not. (Note IPV Prevalence data for 2000/2001 is not available.)
UGANDA PASSED THE “DOMESTIC VIOLENCE ACT” IN 2010. BETWEEN 2000 AND 2011 THERE HAS BEEN A MEANINGFUL DECREASE IN THE NUMBER OF UGANDANS WHO FIND DOMESTIC VIOLENCE ACCEPTABLE. OUR GLOBAL DATA ASSESSMENT HAS INDICATED A LINK BETWEEN SOCIAL ATTITUDES TOWARDS PARTNER VIOLENCE AND PREVALENCE ON A COUNTRY LEVEL. WHAT STANDS OUT IN THIS CHART IS THAT IN SPITE OF THE CHANGES IN UGANDANS’ ATTITUDES THE PREVALENCE RATE OF DOMESTIC VIOLENCE HAS REMAINED STEADY AT ABOUT 60 PERCENT.
We looked at nine different socioeconomic factors from the Uganda DHS dataset – looking particularly for changes over time in their connection to IPV prevalence. Of the nine, only three showed statistically significant changes: Rural vs. Urban residence, Education achieved and Wealth level. No other factors demonstrated significant associations over time. Two highlights are shown below.
Residence Location
Women living in rural areas are at greater risk of experiencing IPV than urban dwelling women in Uganda across both years.
There was a significant drop in IPV rates among rural women from 2006 to 2011.
Wald test results F=230 on 2,8 df with a p-value of <.001
The change in IPV rates over time for urban women is not significant.
Wald Results F=0.03 on 2,8 df with a p-value of .84
The bar chart below captures these statistics visually, showing the IPV prevalence rate for both rural and urban dwellers in 2006 and 2011.
WHILE UGANDA’S NATIONAL PREVALENCE RATE OF DOMESTIC VIOLENCE HASN’T MOVED MUCH BETWEEN 2006 AND 2011, IT IS INTERESTING TO NOTE THE DROP IN RATE OF REPORTED DOMESTIC VIOLENCE IN RURAL AREAS -- FROM 62 PERCENT 2006 TO 52 PERCENT IN 2011. SOMETHING IS HAVING AN EFFECT.
Wealth
Women from the upper levels of wealth were significantly less likely to report experiencing IPV than women at the lower levels of wealth.
This gap widened between 2006 and 2011. In 2006 there was an 18-point difference in the richest women’s prevalence rates and the poorest women’s rates. By 2011 there is a 24-point difference between the richest and poorest women’s rates of IPV experience.
This difference is statistically significant.
Wald test results F-56.64 on 5, 17 df with a p-value of <.001
The bar chart below captures the data and trend across all 5 wealth groups between 2006 and 2011.
CHAPTER 6
SHARING THE DATA
OVERVIEW
One of Orb Media’s primary goals is to establish a new approach for using data together with journalism. As much as we strive and invest in making our stories about all people of the world, practical realities of time and budget limit how much we can do. We can only visit so many countries, and we can only analyze a subset of the data.
The native, raw data we used is available for download directly from the organizations and sites listed in the Original Data Sources chapter. Those datasets are the primary sources, and those sites contain critical information on understanding the data.
We wanted to go further in sharing, however, and add a bit more value. We made a conscious decision on this story – with a topic that is immensely personal and important – to share our processed data so that others could build on what we have done. We want to enable all of us - the global community – to gain further insight into individual countries or regions of interest.
Anyone who works with data – from the novice playing with spreadsheets to the seasoned professional writing custom statistical and machine learning scripts - knows that data cleaning and preparation are often the most time-consuming steps in the data process. To help alleviate this burden – to encourage more people to download and use this data – Orb Media is making our cleaned data available for download.
In the Download section of this site, you can find cleaned versions of most of our datasets, along with a codebook for each.